Separation of Coexisting Communication and Radar Signals within the Same Frequency Band Using Deep Learning
Vol. 50, No. 4, pp. 611-615, Apr. 2025

-
Deep Learning Communication Signal Radar signal Interference Frequency Overlap Signal separation U-net Conv-TasNet
PDF Full-Text
Abstract
Statistics
Cumulative Counts from November, 2022
Multiple requests among the same browser session are counted as one view. If you mouse over a chart, the values of data points will be shown.
Multiple requests among the same browser session are counted as one view. If you mouse over a chart, the values of data points will be shown.
|
Cite this article
[IEEE Style]
S. Jung and H. Nam, "Separation of Coexisting Communication and Radar Signals within the Same Frequency Band Using Deep Learning," The Journal of Korean Institute of Communications and Information Sciences, vol. 50, no. 4, pp. 611-615, 2025. DOI: 10.7840/kics.2025.50.4.611.
[ACM Style]
Suk-hyun Jung and Hae-woon Nam. 2025. Separation of Coexisting Communication and Radar Signals within the Same Frequency Band Using Deep Learning. The Journal of Korean Institute of Communications and Information Sciences, 50, 4, (2025), 611-615. DOI: 10.7840/kics.2025.50.4.611.
[KICS Style]
Suk-hyun Jung and Hae-woon Nam, "Separation of Coexisting Communication and Radar Signals within the Same Frequency Band Using Deep Learning," The Journal of Korean Institute of Communications and Information Sciences, vol. 50, no. 4, pp. 611-615, 4. 2025. (https://doi.org/10.7840/kics.2025.50.4.611)
Vol. 50, No. 4 Index
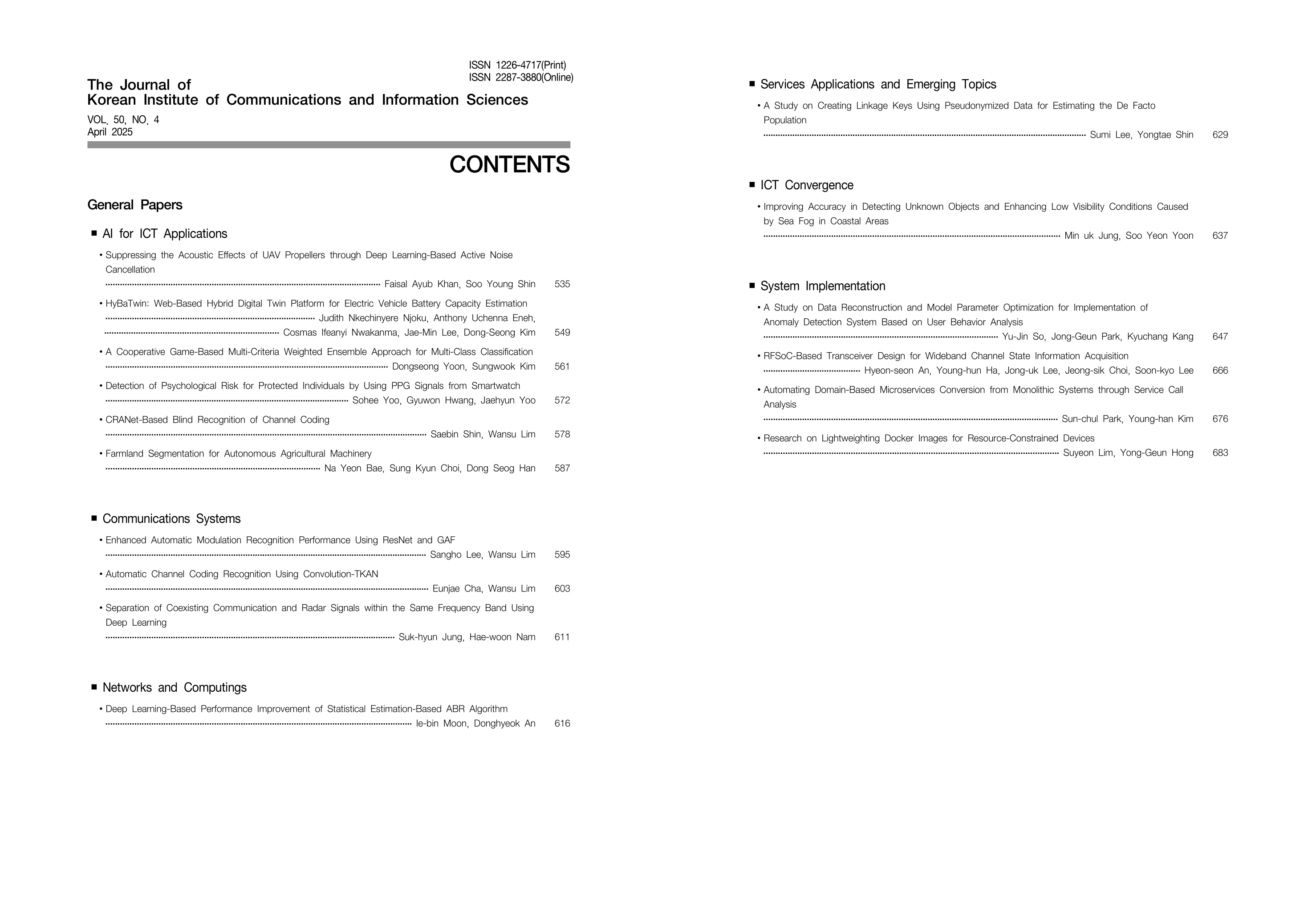