A Study on Highway Driving Decision Making with Hybrid Action for Autonomous Vehicles Using Deep Reinforcement Learning
Vol. 49, No. 12, pp. 1671-1684, Dec. 2024

PDF Full-Text
Abstract
Statistics
Cumulative Counts from November, 2022
Multiple requests among the same browser session are counted as one view. If you mouse over a chart, the values of data points will be shown.
Multiple requests among the same browser session are counted as one view. If you mouse over a chart, the values of data points will be shown.
|
Cite this article
[IEEE Style]
S. Kim, K. Shin, J. Jeon, J. Bang, J. Kim, S. Jung, "A Study on Highway Driving Decision Making with Hybrid Action for Autonomous Vehicles Using Deep Reinforcement Learning," The Journal of Korean Institute of Communications and Information Sciences, vol. 49, no. 12, pp. 1671-1684, 2024. DOI: 10.7840/kics.2024.49.12.1671.
[ACM Style]
Seongjun Kim, Kyu-min Shin, Jun-seo Jeon, Ji-yoon Bang, Junyoung Kim, and Soyi Jung. 2024. A Study on Highway Driving Decision Making with Hybrid Action for Autonomous Vehicles Using Deep Reinforcement Learning. The Journal of Korean Institute of Communications and Information Sciences, 49, 12, (2024), 1671-1684. DOI: 10.7840/kics.2024.49.12.1671.
[KICS Style]
Seongjun Kim, Kyu-min Shin, Jun-seo Jeon, Ji-yoon Bang, Junyoung Kim, Soyi Jung, "A Study on Highway Driving Decision Making with Hybrid Action for Autonomous Vehicles Using Deep Reinforcement Learning," The Journal of Korean Institute of Communications and Information Sciences, vol. 49, no. 12, pp. 1671-1684, 12. 2024. (https://doi.org/10.7840/kics.2024.49.12.1671)
Vol. 49, No. 12 Index
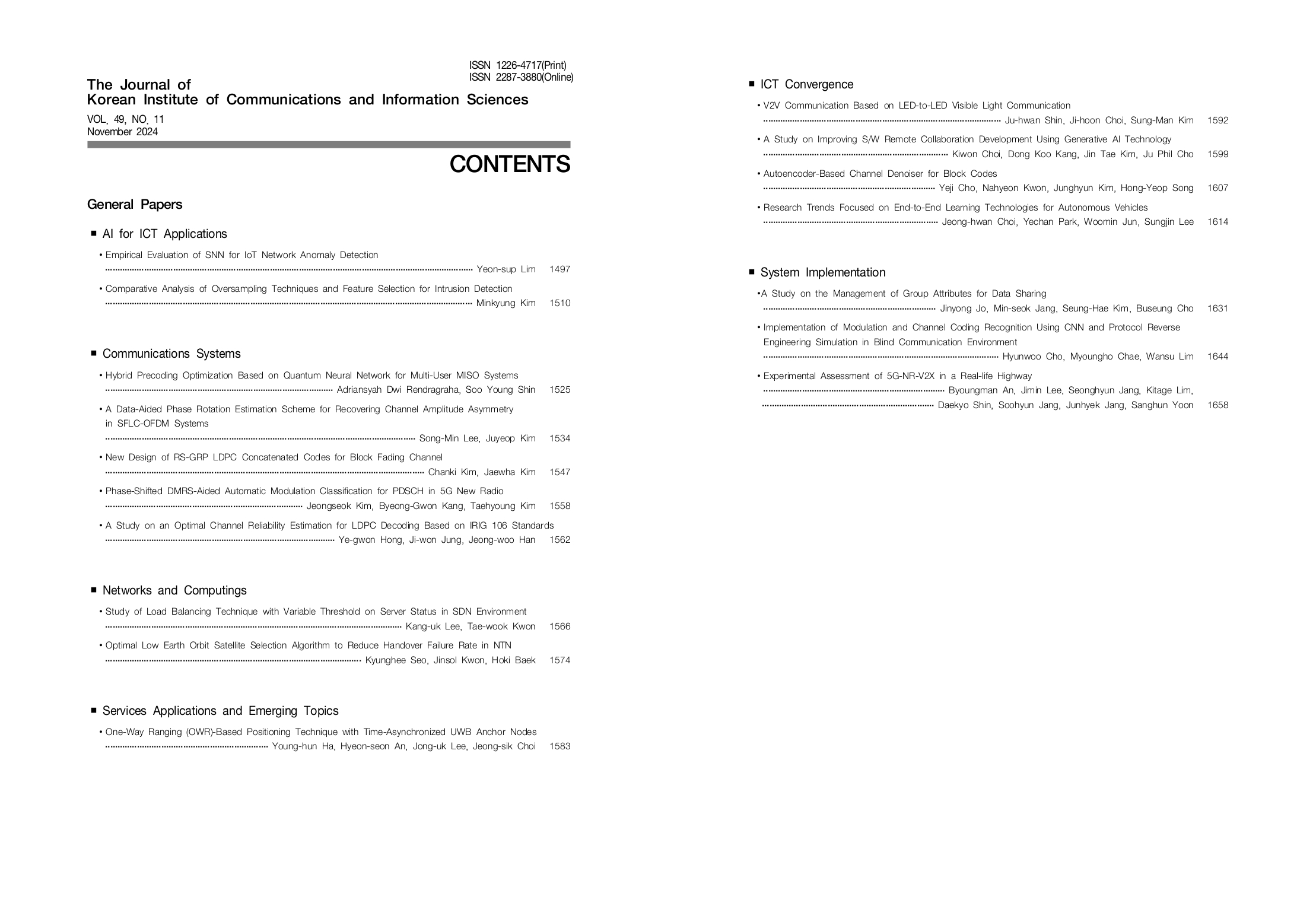