A Survey on Weapon-Target Assignment for Realistic Battlefield Environments: From Exact Algorithm to Deep Reinforcement Learning
Vol. 50, No. 2, pp. 205-216, Feb. 2025

-
Military decision-making Weapon-target assignment Intelligent command decision Deep Reinforcement Learning Heuristic Algorithm
PDF Full-Text
Abstract
Statistics
Cumulative Counts from November, 2022
Multiple requests among the same browser session are counted as one view. If you mouse over a chart, the values of data points will be shown.
Multiple requests among the same browser session are counted as one view. If you mouse over a chart, the values of data points will be shown.
|
Cite this article
[IEEE Style]
C. Eom, J. Lee, M. Kwon, "A Survey on Weapon-Target Assignment for Realistic Battlefield Environments: From Exact Algorithm to Deep Reinforcement Learning," The Journal of Korean Institute of Communications and Information Sciences, vol. 50, no. 2, pp. 205-216, 2025. DOI: 10.7840/kics.2025.50.2.205.
[ACM Style]
Chanin Eom, Jaehwi Lee, and Minhae Kwon. 2025. A Survey on Weapon-Target Assignment for Realistic Battlefield Environments: From Exact Algorithm to Deep Reinforcement Learning. The Journal of Korean Institute of Communications and Information Sciences, 50, 2, (2025), 205-216. DOI: 10.7840/kics.2025.50.2.205.
[KICS Style]
Chanin Eom, Jaehwi Lee, Minhae Kwon, "A Survey on Weapon-Target Assignment for Realistic Battlefield Environments: From Exact Algorithm to Deep Reinforcement Learning," The Journal of Korean Institute of Communications and Information Sciences, vol. 50, no. 2, pp. 205-216, 2. 2025. (https://doi.org/10.7840/kics.2025.50.2.205)
Vol. 50, No. 2 Index
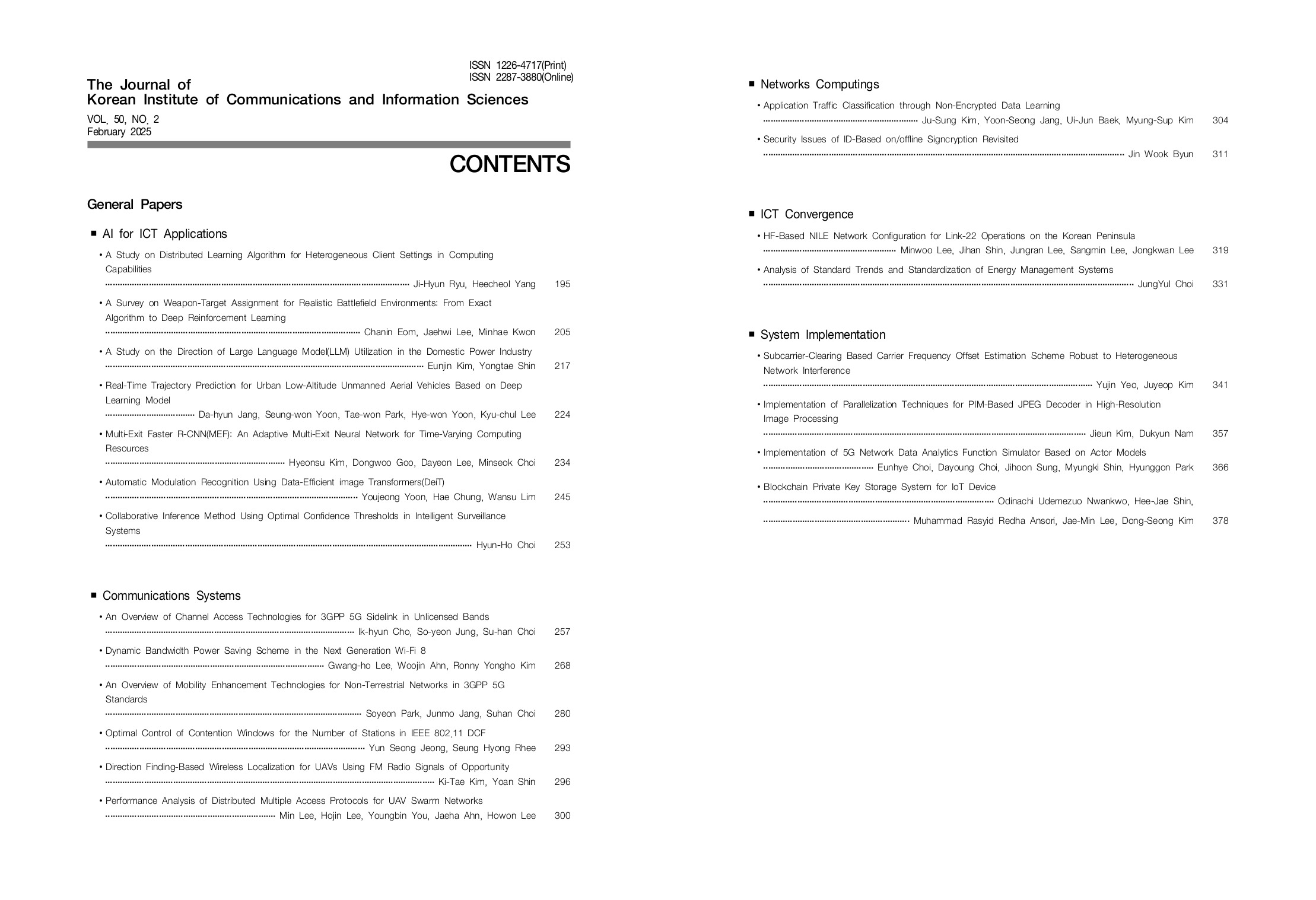