A Survey on Hyperparameter Optimization in Machine Learning
Vol. 48, No. 6, pp. 733-747, Jun. 2023

Abstract
Statistics
Cumulative Counts from November, 2022
Multiple requests among the same browser session are counted as one view. If you mouse over a chart, the values of data points will be shown.
Multiple requests among the same browser session are counted as one view. If you mouse over a chart, the values of data points will be shown.
|
Cite this article
[IEEE Style]
J. Won, J. Shin, J. Kim, J. Lee, "A Survey on Hyperparameter Optimization in Machine Learning," The Journal of Korean Institute of Communications and Information Sciences, vol. 48, no. 6, pp. 733-747, 2023. DOI: 10.7840/kics.2023.48.6.733.
[ACM Style]
Jonghyeon Won, Jongmin Shin, Jae-Ho Kim, and Jang-Won Lee. 2023. A Survey on Hyperparameter Optimization in Machine Learning. The Journal of Korean Institute of Communications and Information Sciences, 48, 6, (2023), 733-747. DOI: 10.7840/kics.2023.48.6.733.
[KICS Style]
Jonghyeon Won, Jongmin Shin, Jae-Ho Kim, Jang-Won Lee, "A Survey on Hyperparameter Optimization in Machine Learning," The Journal of Korean Institute of Communications and Information Sciences, vol. 48, no. 6, pp. 733-747, 6. 2023. (https://doi.org/10.7840/kics.2023.48.6.733)
Vol. 48, No. 6 Index
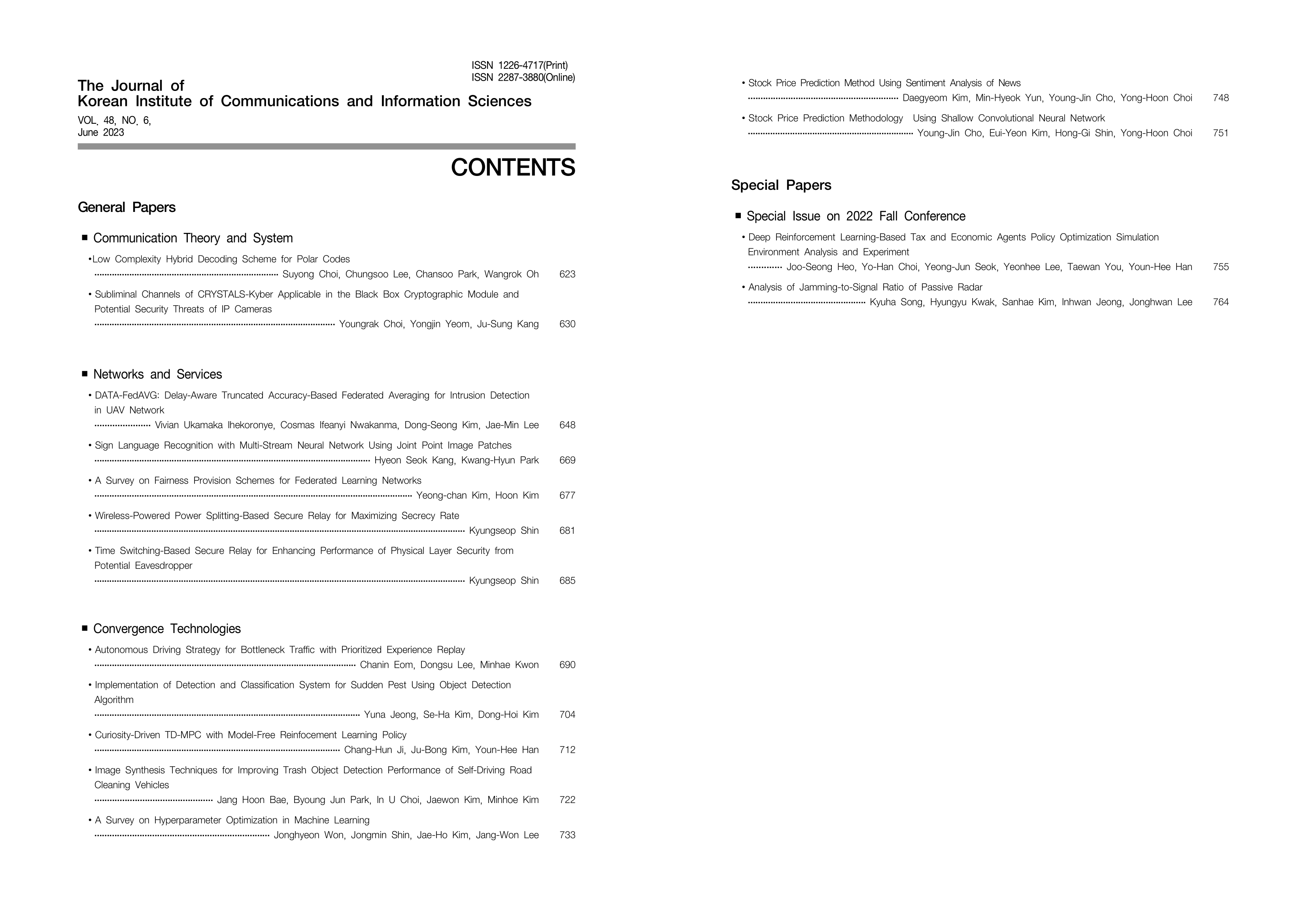