Two-Fold Differentially Private Mechanism for Big Data Analysis
Vol. 49, No. 3, pp. 393-400, Mar. 2024

PDF Full-Text
Abstract
Statistics
Cumulative Counts from November, 2022
Multiple requests among the same browser session are counted as one view. If you mouse over a chart, the values of data points will be shown.
Multiple requests among the same browser session are counted as one view. If you mouse over a chart, the values of data points will be shown.
|
Cite this article
[IEEE Style]
A. Utaliyeva and Y. Choi, "Two-Fold Differentially Private Mechanism for Big Data Analysis," The Journal of Korean Institute of Communications and Information Sciences, vol. 49, no. 3, pp. 393-400, 2024. DOI: 10.7840/kics.2024.49.3.393.
[ACM Style]
Assem Utaliyeva and Yoon-Ho Choi. 2024. Two-Fold Differentially Private Mechanism for Big Data Analysis. The Journal of Korean Institute of Communications and Information Sciences, 49, 3, (2024), 393-400. DOI: 10.7840/kics.2024.49.3.393.
[KICS Style]
Assem Utaliyeva and Yoon-Ho Choi, "Two-Fold Differentially Private Mechanism for Big Data Analysis," The Journal of Korean Institute of Communications and Information Sciences, vol. 49, no. 3, pp. 393-400, 3. 2024. (https://doi.org/10.7840/kics.2024.49.3.393)
Vol. 49, No. 3 Index
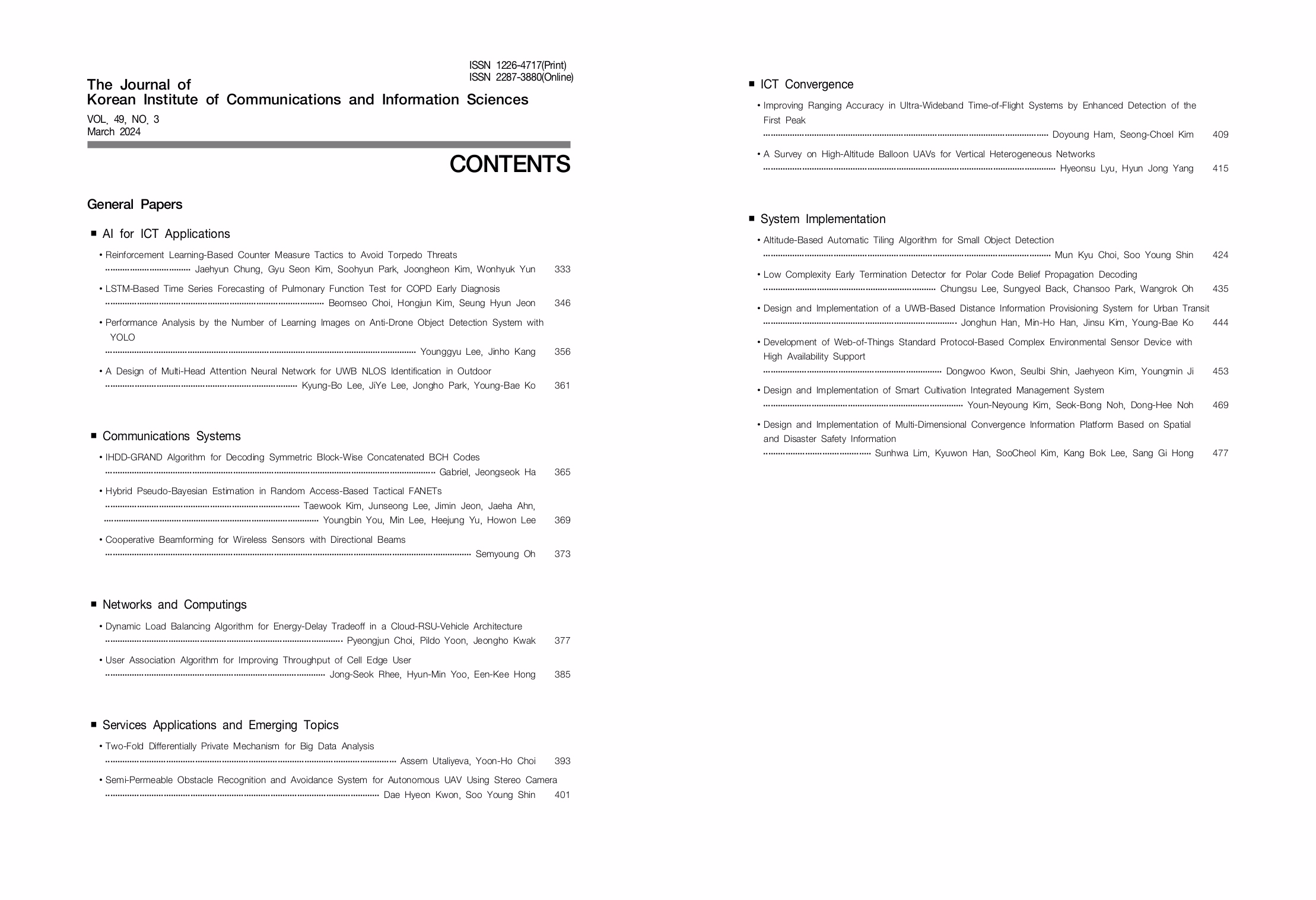