Best Papers Implementation of Music Similarity Analysis System Employing Source Separation and Automatic Transcription Learning
Vol. 48, No. 11, pp. 1500-1508, Nov. 2023

PDF Full-Text
Abstract
Statistics
Cumulative Counts from November, 2022
Multiple requests among the same browser session are counted as one view. If you mouse over a chart, the values of data points will be shown.
Multiple requests among the same browser session are counted as one view. If you mouse over a chart, the values of data points will be shown.
|
Cite this article
[IEEE Style]
Y. Gu and J. Lee, "Implementation of Music Similarity Analysis System Employing Source Separation and Automatic Transcription Learning," The Journal of Korean Institute of Communications and Information Sciences, vol. 48, no. 11, pp. 1500-1508, 2023. DOI: 10.7840/kics.2023.48.11.1500.
[ACM Style]
Yeonwoo Gu and Jaeho Lee. 2023. Implementation of Music Similarity Analysis System Employing Source Separation and Automatic Transcription Learning. The Journal of Korean Institute of Communications and Information Sciences, 48, 11, (2023), 1500-1508. DOI: 10.7840/kics.2023.48.11.1500.
[KICS Style]
Yeonwoo Gu and Jaeho Lee, "Implementation of Music Similarity Analysis System Employing Source Separation and Automatic Transcription Learning," The Journal of Korean Institute of Communications and Information Sciences, vol. 48, no. 11, pp. 1500-1508, 11. 2023. (https://doi.org/10.7840/kics.2023.48.11.1500)
Vol. 48, No. 11 Index
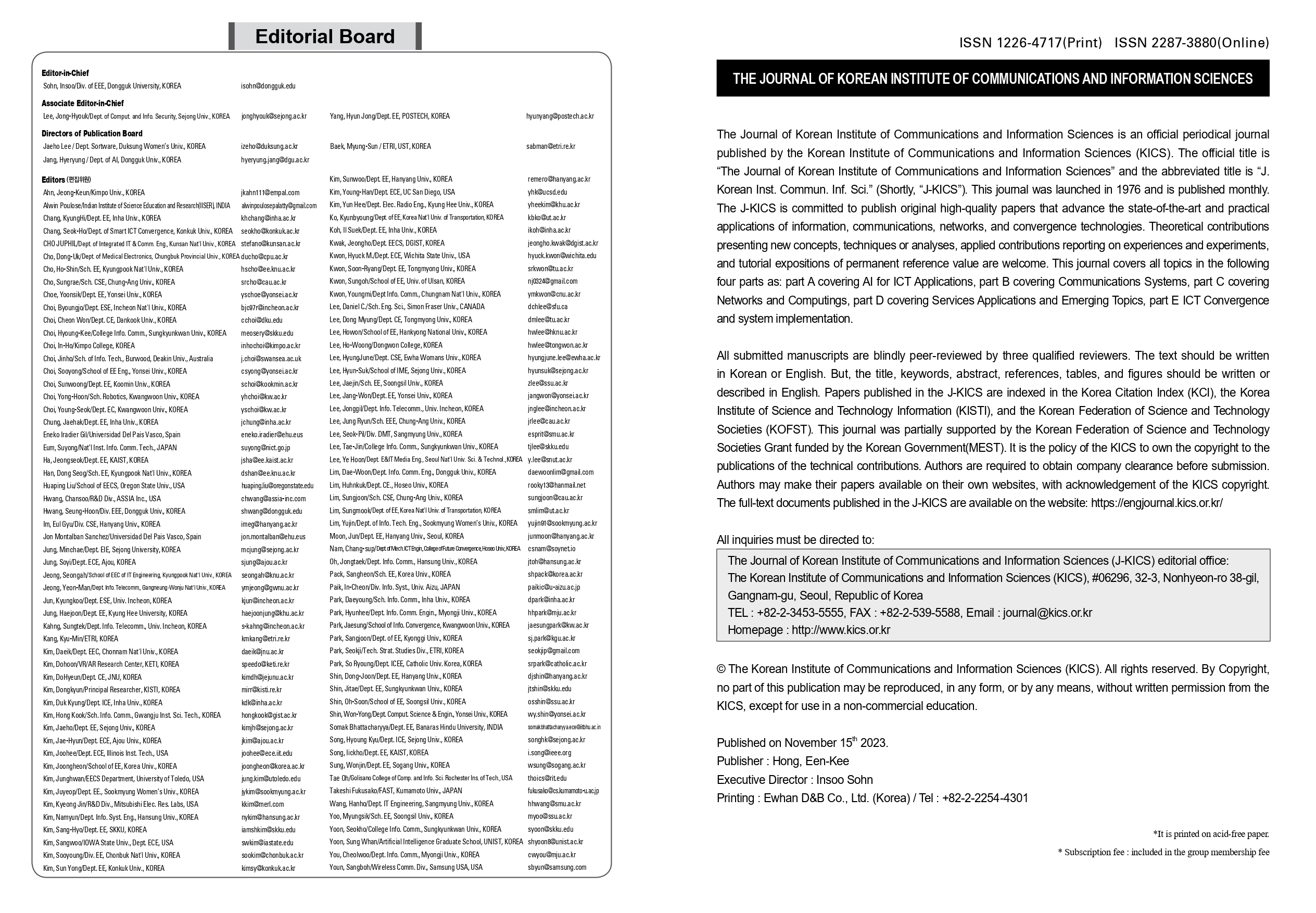