Enhancing Chest X-ray Image Super-Resolution through Residual Group and Channel Attention
Vol. 49, No. 5, pp. 762-772, May 2024

-
Chest X-ray super-resolution Remaining Enhanced channel attention block Exponential Liner Unit Structure Similarity Index Multi Structure Similarity Index.
PDF Full-Text
Abstract
Statistics
Cumulative Counts from November, 2022
Multiple requests among the same browser session are counted as one view. If you mouse over a chart, the values of data points will be shown.
Multiple requests among the same browser session are counted as one view. If you mouse over a chart, the values of data points will be shown.
|
Cite this article
[IEEE Style]
A. Khishigdelger, A. Salem, H. S. Kang, "Enhancing Chest X-ray Image Super-Resolution through Residual Group and Channel Attention," The Journal of Korean Institute of Communications and Information Sciences, vol. 49, no. 5, pp. 762-772, 2024. DOI: 10.7840/kics.2024.49.5.762.
[ACM Style]
Anudari Khishigdelger, Ahmed Salem, and Hyun Soo Kang. 2024. Enhancing Chest X-ray Image Super-Resolution through Residual Group and Channel Attention. The Journal of Korean Institute of Communications and Information Sciences, 49, 5, (2024), 762-772. DOI: 10.7840/kics.2024.49.5.762.
[KICS Style]
Anudari Khishigdelger, Ahmed Salem, Hyun Soo Kang, "Enhancing Chest X-ray Image Super-Resolution through Residual Group and Channel Attention," The Journal of Korean Institute of Communications and Information Sciences, vol. 49, no. 5, pp. 762-772, 5. 2024. (https://doi.org/10.7840/kics.2024.49.5.762)
Vol. 49, No. 5 Index
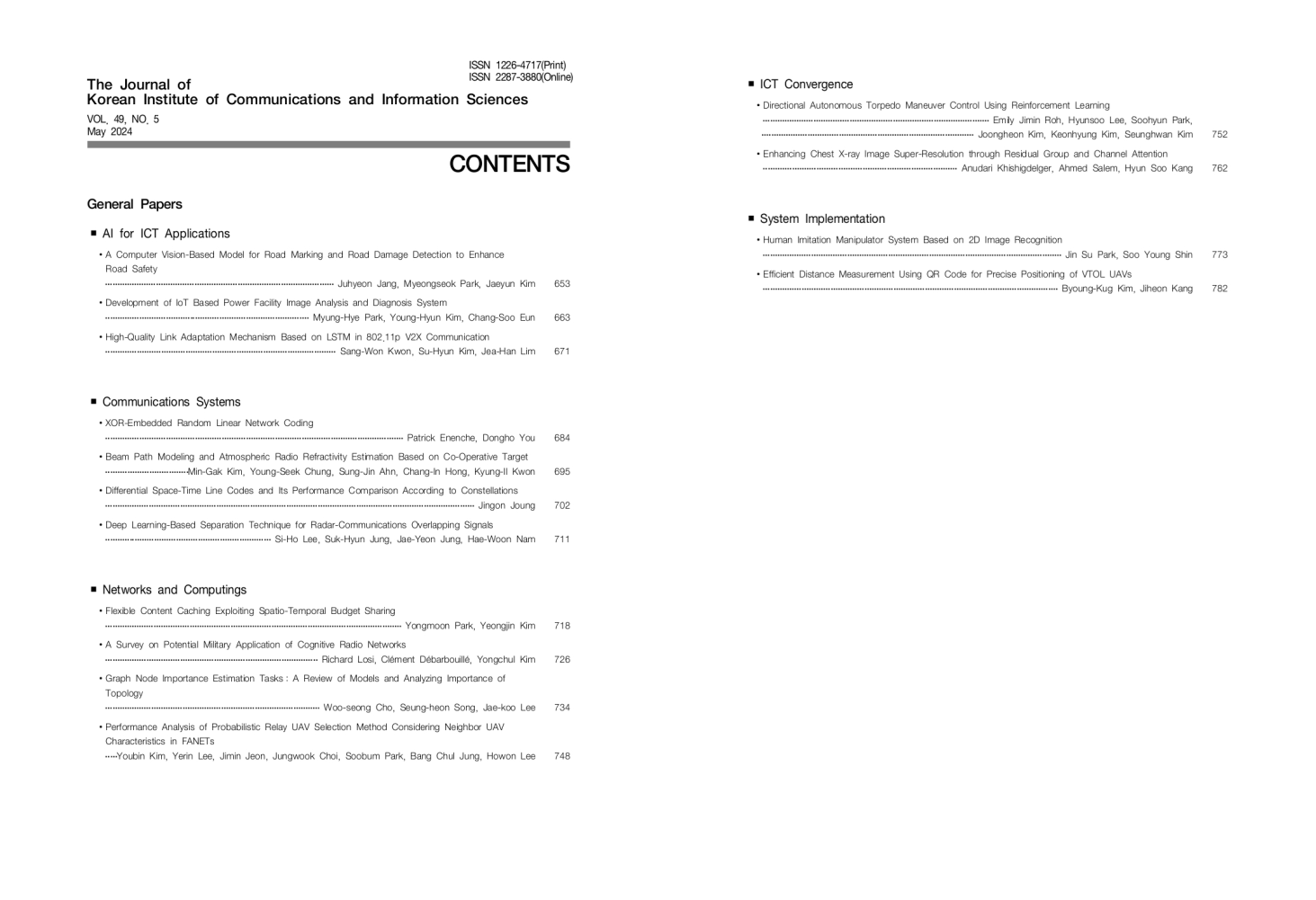